CentralPoint - Evolve Your Data
Artificial Intelligence is rapidly changing how organizations operate and make decisions. CIO's and other company leaders may feel the pressure to take advantage of AI to improve processes, automate operations and reduce costs. The ability of AI to analyze enterprise data sources and automate processes is changing many industries. The fact is AI is deeply dependent upon the quality of data it accesses. Additionally, the integrations and process management sitting inbetween AI and the data sources becomes even more crucial.
According to a recent McKinsey report:
"only about 10% of surveyed companies realize significant financial benefits from AI initiatives." Similarly, a study by Appen suggests that a "lack of high-quality data and organizational challenges are primary barriers to successful AI deployment." Moreover, around 40% of companies remain stuck in preliminary stages of adoption without fully scaling AI projects across their organizations.
"Those who succeed often ensure a robust data foundation and prioritize integrating AI into core business processes"
Read further on how an organization can meet these challenges and what cost effective tools can be utilized to make your AI, Data and Integration Projects successful.
How Does an Organization Pragmatically and Cost Effectively Prepare for AI Projects?
The CentralPoint Platform can function both as a Data Mesh and Data Transformation Tool. Either ingest your data for cleansing or utilize the Data Governance validation rules and workflows over a Data Mesh. Manage your Data Mesh and Data Pipelines while synchronizing disparate systems real-time. Realtime Event and Data Pipeline streams can be managed with Governance rules applied. Enrich and apply rule based meta-data to structured or unstructured data. Dynamic workflows including dynamic document creation is also part of the CentralPoint platform.
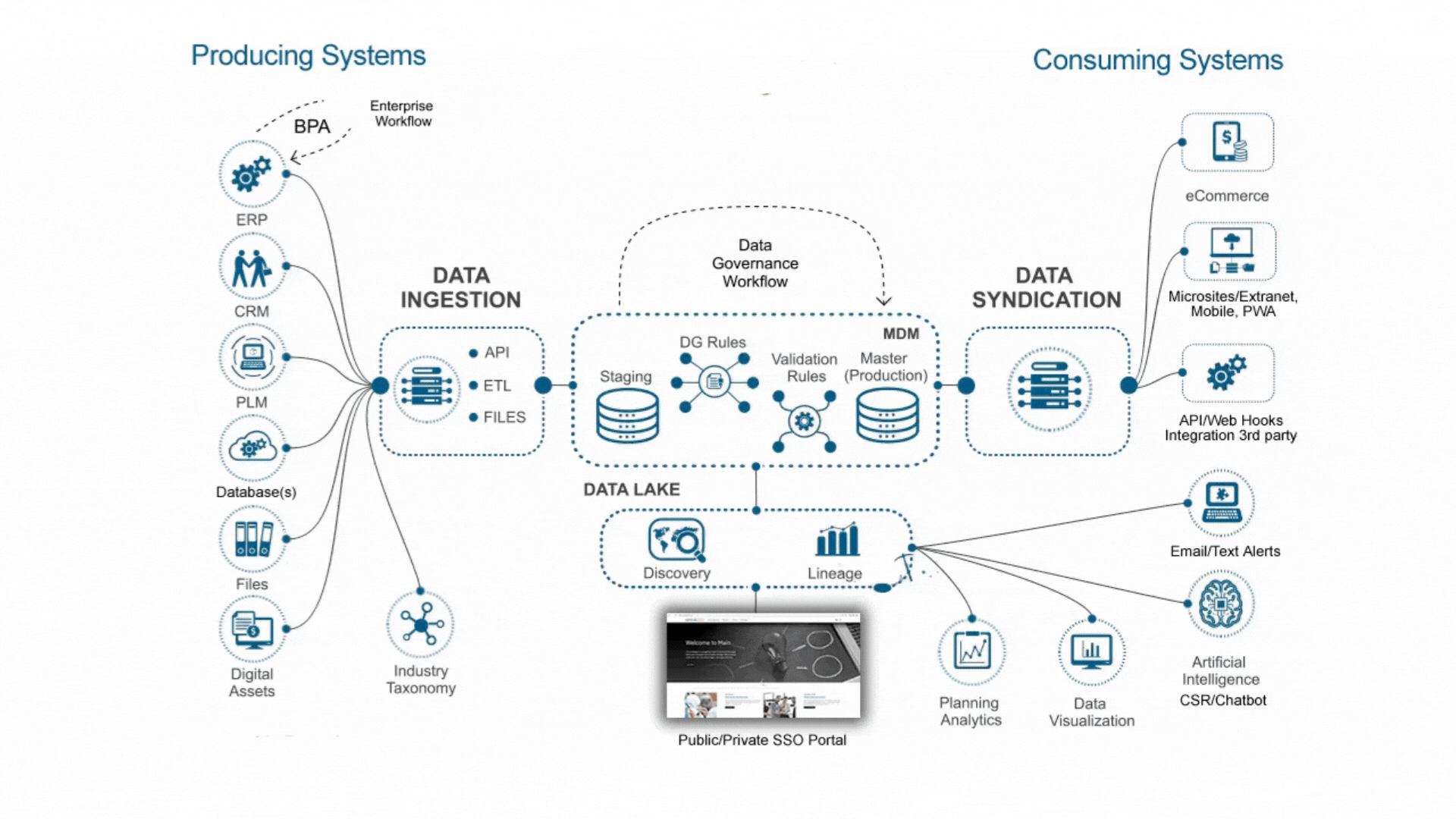
CentralPoint Capabilities and Data Quality Best Practices
Implement Strong Data Streaming and Collection Procedures
Establish strong Data Streaming and collection processes, policies and governance. This includes policies on how data is stored, where it is stored, security, and clear guidelines on how the data is processed. This includes data and integration tools like CentralPoint and Kubeark that capture data and process errors.
Automate Cleansing and Sanitizing Data
Cleansing and sanitizing data involves removing or correcting data that is incorrect, incomplete, does not follow established field definitions, is duplicated or improperly formatted. Automated cleansing includes auto-classification of each record with accurate metadata and taxonomy rules at rest or in the data pipeline.
Use Data Validation Rules
Implementing Data Validation rules at rest and in the streaming Data Pipeline is a proactive approach to ensure the accuracy of data wherever it may be used. Using EssorTec's methods and tools ensures that your data is always reviewed if a validation error is encountered. Automation and workflow routing via dynamic form creation for the Data Product Owner to review and either accept or establish a new rule via a push of the button is part of the EssorTec tools.
Integrate and Mesh Data from Multiple Sources Carefully
When combining data from different sources into a central repository, it is essential to ensure consistency. Additionally, when deploying a data mesh compatibility between systems that use the same data element should be reviewed. This gives rise to the method of Data Product and Data Product Owners. The EssorTec methodology ensures data that is used multiple places is standardized or transformed via a standard transformation. This strategy may include matching data formats, ensuring the data scales and units are uniform. Integration that is supports and is deployed within the Data Ecosystem prevents data conflicts and loss of integrity.
Automate Data and Record Retention Policies
CentralPoint automates your Data and Record Retention Policies - structured or unstructured. CentralPoint can also provide reports on Data that has become stale and needs to be refreshed, replaced or removed. CentralPoint uses scheduled updates as well as check to verify that the new data maintains the same quality standards as the existing data.
Automate Routine Data Quality Audits
Conducting regular audits involves systematically reviewing and checking data for accuracy, completeness, conformance, observability and consistency. This process helps rectify anomalies, duplicates or outdated information before decision making processes are impacted. Routine audits also ensure that data governance processes are being followed and that data management practices are effective.
Deploy Data Governance Practices
Data governance includes policies, standards, and procedures that ensure data is managed appropriately and used for its intended purpose in the organization. This can include Application, System, Roles or Attributes for security access to the data. It includes setting clear roles and responsibilities for data management, establishing the data standards and defining the data access protocols.
Automate Data Standardization Protocols
Standardization protocols ensure that data from various sources is consistent and comparable. This includes adopting common formats, technologies, and units across all data sources. Standardization and the Standardization of Transformation between systems during integration significantly reduces the efforts of data cleaning, migration or archive access.
Enhanced Real-Time Data Processing
With the rise of Real-Time Data Streams, ensuring Quality Data in real-time is crucial. Tools and methods such as EssorTec will be essential for applications requiring immediate insights, such as autonomous vehicles, real-time fraud detection, AI fraud detection and hospitals to name a few.
Cross Domain (Company) Data Quality
The EssorTec tools and methods support Cross Domain Data Quality, Transformation and Integration. This is applicable in companies with autonomous divisions, companies that work closely with sub-contractors, regulatory bodies and cross domain processes. The capability to manage, transform and standardize these integrations will be increasingly important. In effect, data becomes boundless within the established data governance and security rules.
AI Success Requires High Quality Data
High Quality and Governed Data is THE most critical component for the deployment, accuracy and success of AI projects. The future is now and organizations that focus on Data Quality, Governance and deploy the tools to support these efforts will be in a position to drive efficiencies and automation through their enterprise.